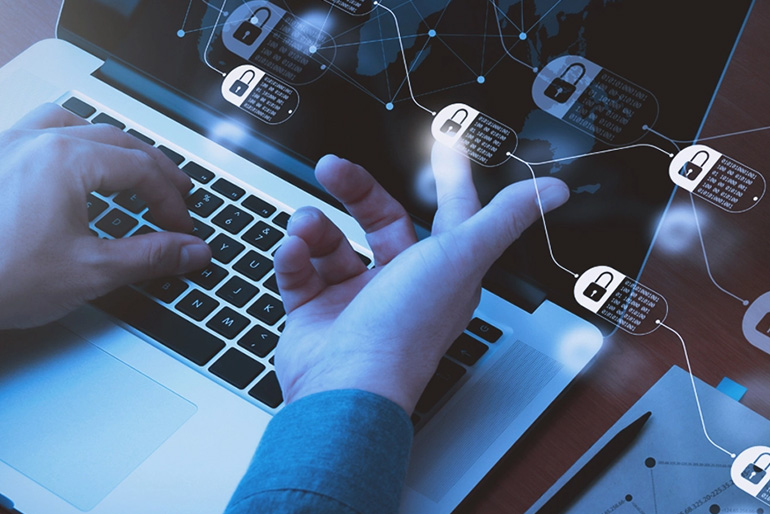
Machine learning is a category of artificial intelligence (AI) that includes the development of statistical and algorithms models, which permits computers to learn via data without being openly programmed. Creating systems with the capability to continuously ameliorate their performance on a given task grounded on the experience attained from the data they’re exposed to is the end of machine learning. This is fulfilled by giving algorithms expansive training on huge data sets, which enables the algorithms to find patterns and connections in the data.
Supervised learning: This involves training a model on a labeled data set, where the correct affair is handed for each input. The algorithm uses this information to learn the relationship between inputs and labor and can also make prognostications on new, unseen data.
Unsupervised learning: This involves training a model on an unlabeled data set where the correct affair isn’t handed. The algorithm must find the structure in the data on its own and is generally used for clustering, dimensionality reduction, and anomaly discovery.
Reinforcement Learning: This involves training an agent to make opinions in a terrain where it receives feedback through prices or corrections. The algorithm uses this feedback to learn the outstanding strategy for maximizing prices over time.
The integration of machine learning and blockchain technology holds a great pledge for the future. Machine learning algorithms can be used to assess the data and induce prognostications grounded on it using a decentralized and secure platform like the blockchain.
One possible area of operation for this integration is in the banking sector, where blockchain technology’s decentralized character and capability to enjoin unauthorized access to sensitive data can help machine learning algorithms descry fraud and plutocrat censoring more efficiently.
Machine learning and blockchain technology can also make a significant difference in force chain operations. While blockchain technology can be used to give openness and responsibility in the force chain, machine learning algorithms can be employed to optimize force chain operations and read demand.
Blockchain technology can enable the secure and private sharing of medical records, while machine learning algorithms can be used to prognosticate complaint outbreaks and enhance patient issues.
The future of machine learning is anticipated to be characterized by uninterrupted advances in algorithms, calculating power, and data vacuity. As machine learning becomes further extensively espoused and integrated into colorful diligence, it has the implicit to greatly impact society in several ways.
Some of the crucial trends and developments in the future of machine learning include
More Automation: As machine learning algorithms progress, they will be suitable to automate a larger range of jobs, taking lower mortal input and boosting productivity.
More Individualized Experiences: Machine learning algorithms will have the capacity to assess and make use of enormous volumes of data to deliver a largely tailored experience, similar to substantiated suggestions and announcements.
Enhanced judgment: As machine learning algorithms get better at making complicated judgments and prognostications, multitudinous businesses will profit from the more precise and effective decisions- timber.
AI ethical advancements: As machine learning becomes more common, there will be a growing emphasis on icing that it’s developed and employed immorally and responsibly, with a focus on securing sequestration and barring impulses in decision-timber.
Interdisciplinary collaboration: Machine learning will decreasingly be used in collaboration with other fields, similar to neuroscience and biology, to drive discoveries and advancements in those areas.
Overall, the future of machine learning holds a great pledge and is anticipated to continue transubstantiating a wide range of diligence, from finance to healthcare, in the coming times.