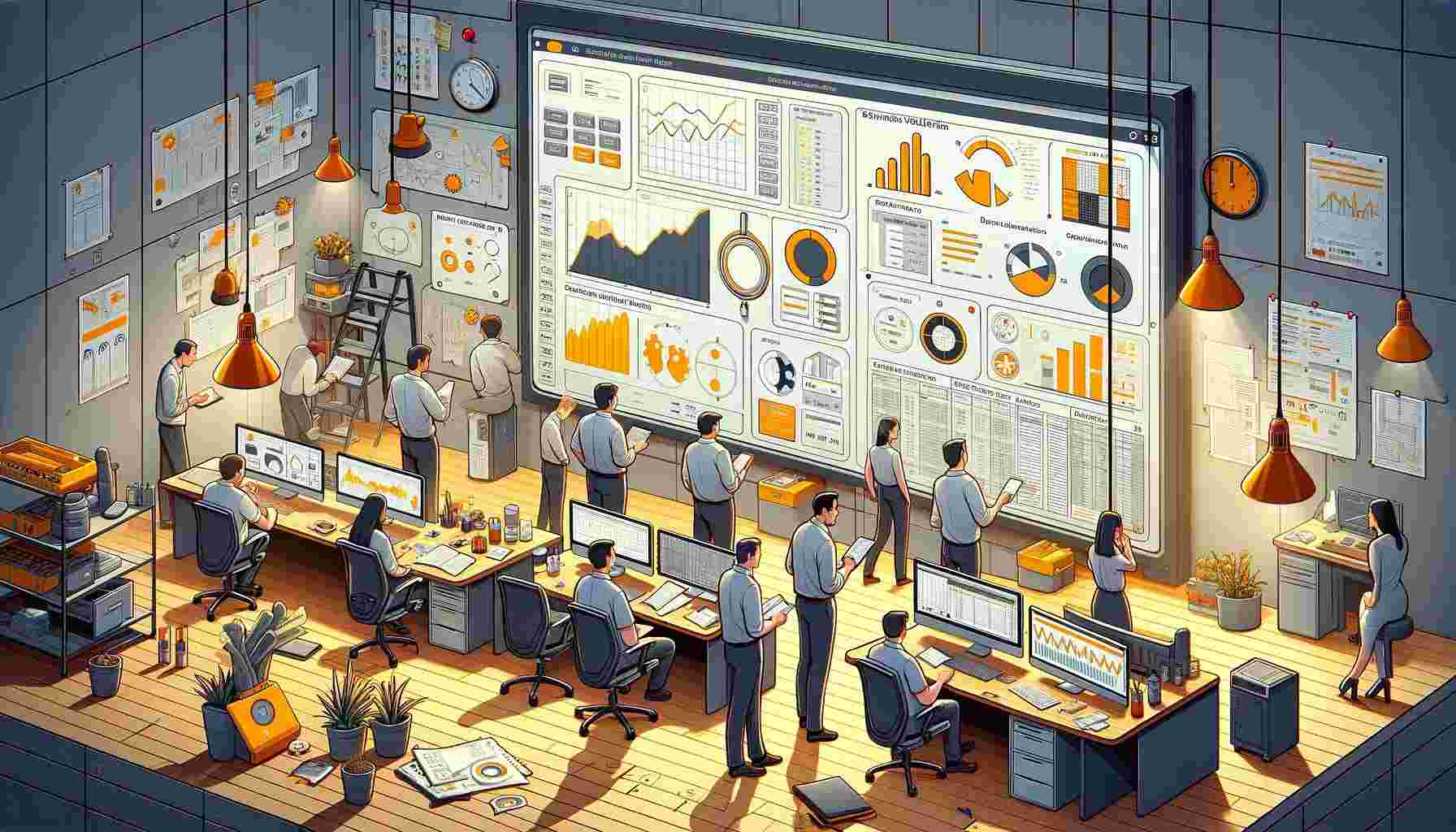
Validation of design is a crucial stage in the product development process, guaranteeing that a product fulfills the planned user requirements and performance criteria under specific operating conditions. Statistical methods and approaches are essential in this phase, offering a quantitative foundation for making well-informed decisions and evaluating the dependability and efficiency of design components. This piece explores the primary statistical tools and techniques employed in design validation and their practical uses.
What does Design Validation entail?
Design validation involves testing and assessing a product design to guarantee that it complies with the designated requirements and operates as intended. This stage is critical in identifying any possible issues or flaws early in the design process, enabling essential modifications to be implemented before mass production. Through comprehensive design validation, companies can minimize the likelihood of expensive errors and guarantee that their products fulfill customer expectations.
Importance of Statistics in Design Validation
Design validation relies on statistics as it provides a methodical way to gather, analyze, and interpret data. This is crucial for confirming design assumptions and meeting regulatory standards. In sectors like pharmaceuticals, automotive, and aerospace, where safety and effectiveness are of utmost importance, statistical validation aids in comprehending the variability in design performance and ensuring consistent adherence to predetermined criteria.
The Benefits of Design Validation
It offers a multitude of benefits for businesses, including:
- Improved product quality and reliability
- Enhanced customer satisfaction
- Reduced time-to-market for products
- Minimized risk of product failure
- Increased profitability through higher sales
- Competitive advantage in the market
By investing in design validation, companies can optimize their product development processes, minimize risks, and ultimately drive business success. These benefits extend beyond cost savings and can significantly impact overall business performance.
Key Statistical Tools and Techniques
1. Hypothesis Testing
Hypothesis testing is the go-to method when making decisions about a population’s properties based on sample data. Design validation plays a crucial role in determining whether a design change has significantly enhanced the product or if any variances are due to random variation. Various tests, such as t-tests, chi-square tests, and ANOVA, are available, and their applicability depends on the type of data and the specific hypothesis being tested.
2. Regression Analysis
Regression analysis is all about modeling the relationship between a dependent variable and one or more independent variables. Design validation proves invaluable for predicting product performance under different conditions and quantifying the impact of various design parameters on the outcome. This, in turn, aids in optimizing the design to meet the required specifications.
3. Design of Experiments (DOE)
DOE is a structured approach for identifying the relationship between process factors and output. In design validation, it efficiently examines the impact of multiple variables on performance and determines the best design settings. Commonly used techniques include factorial designs, response surface methodology, and Taguchi methods.
4. Reliability testing
Reliability testing evaluates a product’s performance over time under normal usage conditions. Methods such as life data analysis, Weibull analysis, and accelerated life testing are employed to estimate product lifetime and failure rates and identify potential failure modes before the product is released to the market.
5. Monitoring process stability and performance
Monitoring process stability and performance during the prototype phase, control charts are utilized in validation to detect when a process experiences unwanted design variations, ensuring consistent quality and performance.
6. Monte Carlo simulations
Utilized in this validation, Monte Carlo simulations employ randomness to address problems that may be deterministic in principle, allowing for the modeling and understanding of variability in product performance, particularly in complex systems where analytical solutions are not possible.
Applications in Industry
Regression analysis and DOE are utilized in the automotive industry to validate crash safety designs and enhance fuel efficiency. Electronics rely on reliability testing and Monte Carlo simulations to guarantee product performance in diverse environmental conditions. In pharmaceuticals, hypothesis testing and control charts are crucial in ensuring the effectiveness and safety of new drugs for mass production.
Cost-benefit analysis
When assessing the cost-benefit analysis of design validation, it’s important to take into account the following elements:
- The expense of implementing validation procedures
- Potential cost savings from preventing product defects and recalls
- Influence on brand reputation and customer loyalty
- Competitive edge achieved through higher product quality
- Overall return on investment (ROI) for validation activities
By considering these factors and conducting a thorough cost-benefit analysis, companies can make well-informed decisions regarding the importance of validation in their product development approach.
Sum Up
In conclusion, statistical tools and techniques are essential for the design validation process, offering a robust methodology to assess and ensure the safe and effective performance of products. As technology and product complexity advance, the significance of statistics in design validation becomes increasingly important, fostering innovation while upholding compliance and quality in product development.